Introduction to AI: Unleashing the Power of Artificial Intelligence
Power of Artificial Intelligence, or Power of AI, has been making waves across industries and transforming the way we live and work in an era defined by technology developments. It’s an issue that has piqued people’s interest and curiosity, as well as some anxiety. What precisely is artificial intelligence, and what does it signify for our future? In this blog, we’ll go on a trip to debunk AI and discover its enormous potential.
What is Artificial Intelligence?
At its core, Artificial Intelligence is the branch of computer science concerned with developing machines capable of executing activities that would normally need human intelligence. Problem-solving, learning from experience, interpreting natural language, identifying patterns, and making decisions are examples of these tasks. AI systems strive to mimic human cognitive functions such as reasoning, problem solving, perception, and language comprehension.
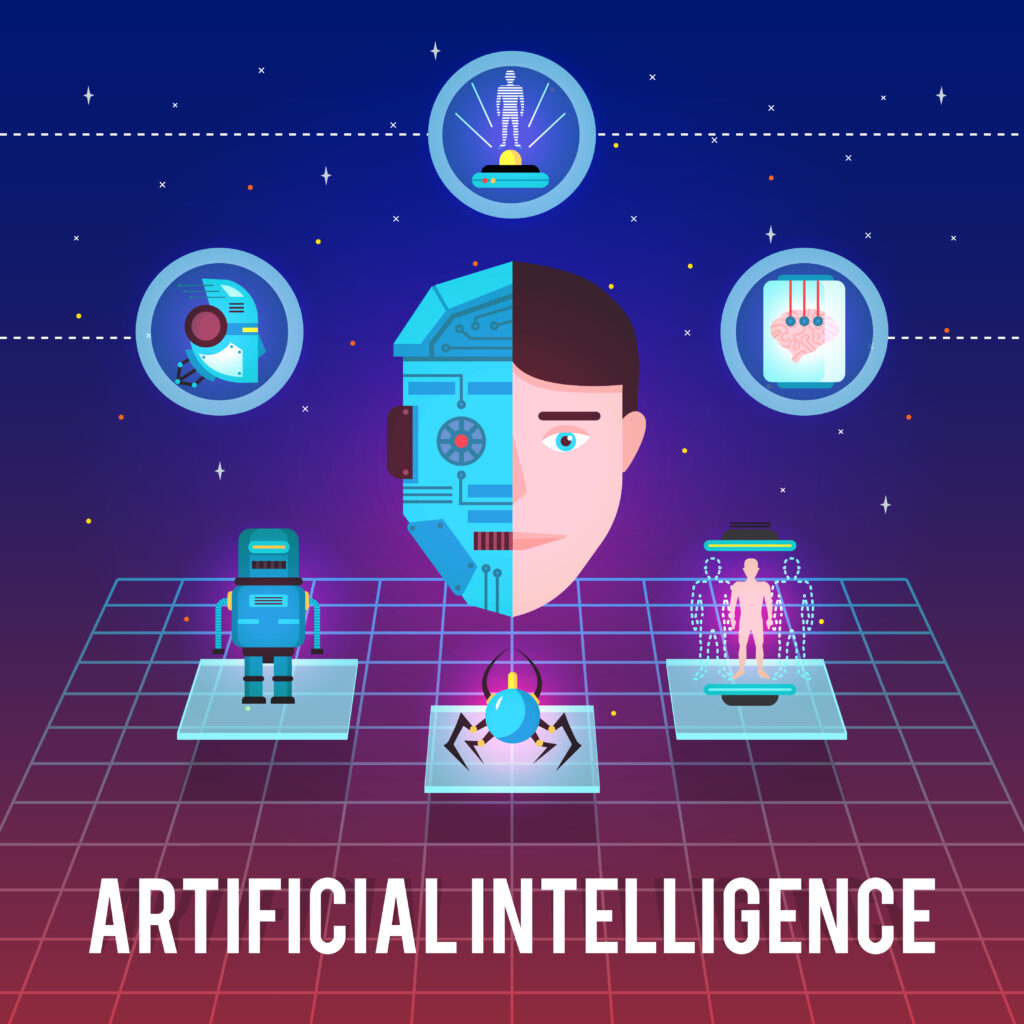
Types of AI
- Narrow or Weak AI
Narrow AI, commonly referred to as Weak AI, is intended to do certain jobs or address specific problems. It works inside a specific domain and excels at tasks for which it has been programmed. Voice assistants like Siri or Alexa, chatbots for customer support, and recommendation algorithms used by streaming platforms are all examples of Narrow AI.
- General or Strong AI
A hypothetical AI system with human-like intellect is represented by General AI, often known as Strong AI or AGI (Artificial General intellect). It would be able to understand, acquire, and apply information across a wide range of tasks, much like a human. We currently have Narrow AI systems, and researchers’ long-term goal is to construct General AI.
The Building Blocks of AI
- Machine Intelligence
Machine Learning is a subfield of artificial intelligence that focuses on allowing machines to learn from data. Instead of explicit programming, ML algorithms make predictions or judgments using patterns and statistical techniques. Linear regression, decision trees, and neural networks are examples of common ML algorithms.
- Deep Learning
Deep Learning is a subfield of machine learning that uses artificial neural networks inspired by the structure of the human brain. Deep learning has transformed AI by allowing it to excel at tasks such as image and speech recognition. Deep learning architectures that are popular include Convolutional Neural Networks (CNNs) and Recurrent Neural Networks (RNNs).
- NLP (Natural Language Processing)
NLP is a subfield of artificial intelligence that focuses on teaching computers to understand, interpret, and generate human language. It provides electricity to applications like language translation, sentiment analysis, and chatbots.
- computer vision
Computer Vision enables robots to interpret and comprehend visual information from their surroundings, making it critical for tasks such as picture identification, object tracking, and autonomous vehicles.
Power of AI Applications
Healthcare: AI is applied in medical image analysis, drug research, and disease outbreak prediction.
Finance: AI helps with algorithmic trading, fraud detection, and credit rating.
Retail: AI is used in e-commerce platforms for product recommendations and supply chain optimization.
Autonomous vehicles are an excellent illustration of AI in action.
Streaming services employ AI to promote content, and AI-generated art is becoming more popular.
The AI Future
As AI advances, its impact on society will only expand and power of AI increases. While technology has enormous potential for beneficial transformation, it also raises significant ethical and societal concerns. In order to shape our AI-powered future, we must strike a balance between innovation and responsible AI development.
Conclusion
To summarize, Power of AI is a disruptive force that is already transforming our environment. Understanding the principles of AI is a valuable step in navigating our increasingly AI-driven world, whether you’re an AI enthusiast or simply interested about the subject.
- You might be interested in next read
- Machine Learning Introduction